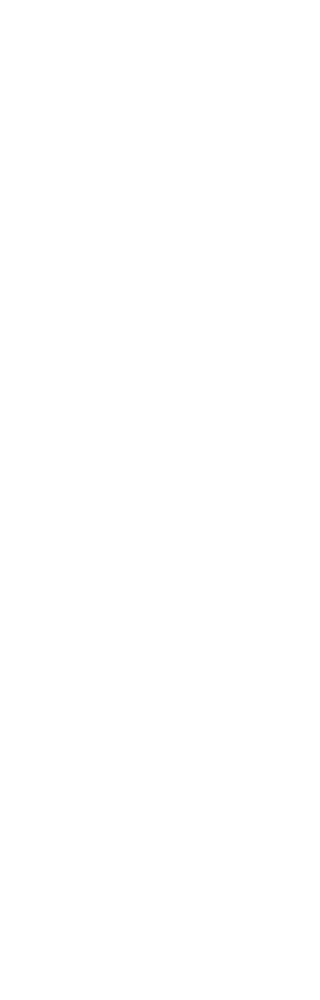
Identifying cancer driver genes from functional genomics screens.
Togar T, Desai S, Mishra R, Terwadkar P, Ramteke M, Ranjan M, Kawle D, Sahoo B, Pal A, Upadhyay P, Dutt A.RNAi screens are robust tools to identify oncogenic dependencies. However, screen identifies a large number of hits which often makes identification of driver genes difficult, unless a secondary screen is set up and targets are validated by functional assays. Studies have suggested that integration of genomics data can help identify potential leads from the screen. Although, several computational tools are available to predict the cancer essential genes by combing the available genomics data, simple tools and scoring systems for easy usage by a functional biologist are lacking. The approach of combining genomics and functional genomics data can assist in identifying driver genes by reducing false positives.
In this manuscript, we describe a simple scoring based approach ‘DepRanker’, which is specifically designed to integrate genomics data such as copy number and gene expression data along with the processed output of a pooled RNAi screen to identify cancer dependency genes. Here, we performed pooled shRNA screen targeting human kinases and additional genes in head and neck cancer cell line AW13516 and integrated genomic features using our in-house integrative scoring system - DepRanker to calculate an impact score (IS) that could predict the potential cancer driver genes from the screen. An example screen output dataset, genomics data and software set-up instructions have been provided at https://github.com/sanket-desai/depranker-git for usage of the software. We further functionally validated the predicted target genes by inhibitor assays and knockdown approach and identified AURKB and TK1 as potential driver kinases conferring oncogenic dependency on these cells.
In summary, we present a simple approach of integrating the genomic data for the cell lines on which pooled RNAi screen is carried out using simplified scoring system that can be readily used by a functional biologist to predict potential cancer essential genes. DepRanker doesn’t necessitate intense computational infrastructure and can be run without specialised and advanced computational expertise of the researchers.
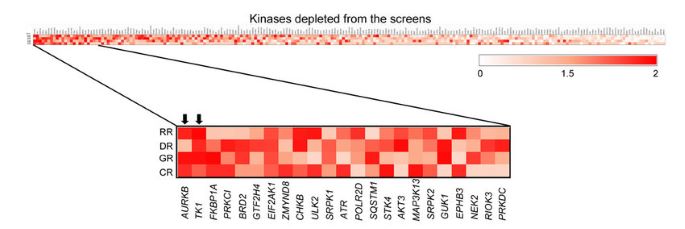